Accelerating Spectral Normalization for Enhancing Robustness of Deep Neural Networks
- Jaden Pan
- May 24, 2022
- 1 min read
Deep neural networks (DNNs) play an important role in machine learning due to its outstanding performance compared to other alternatives. However, DNNs are usually not suitable for safety-critical applications since DNNs can be easily fooled by well-crafted adversarial examples. To address this issue, spectral normalization (SN) technique was proposed to counter adversarial attacks, which ensures that the trained model has low sensitivity towards the disturbance of input samples. Unfortunately, this strategy requires exact computation of spectral norm, which is computation intensive and impractical for large-scale networks. In this paper, we introduce an acceleration technique for spectral normalization based on Fourier transform and layer separation. The proposed method provides DNNs with promising security protection while maintaining minimized time cost, which turns SN from a theoretically feasible approach to a practically useful framework. Experimental evaluation using autonomous systems demonstrates that our acceleration method is able to significantly improve both time efficiency (up to 60%) and model robustness (61% on average) compared with the state-of-the-art spectral normalization in real-world applications.
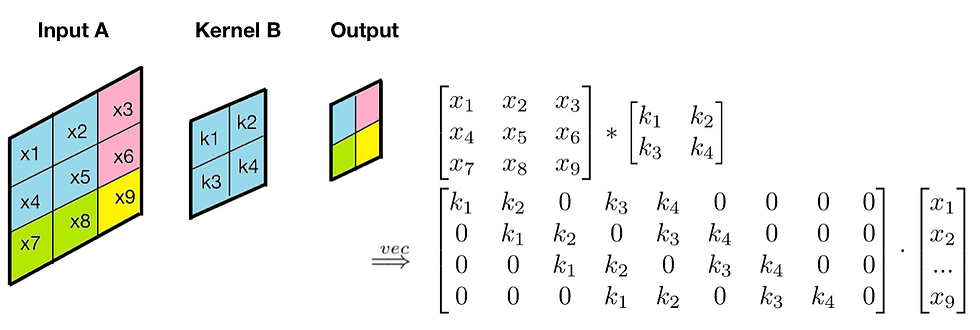

https://ieeexplore.ieee.org/abstract/document/9516747
Comments